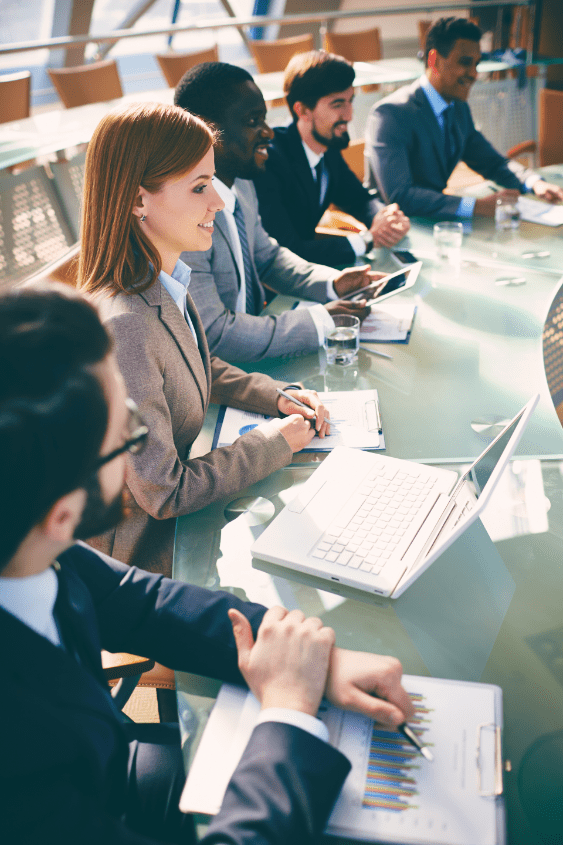
Course Description
This course covers methods and practices to implement data engineering solutions by using Microsoft Fabric. Students will learn how to design and develop effective data loading patterns, data architectures, and orchestration processes. Objectives for this course include ingesting and transforming data and securing, managing, and monitoring data engineering solutions. This course is designed for experienced data professionals skilled at data integration and orchestration, such as those with the DP-203: Azure Data Engineer certification.
Audience Profile
This audience for this course is data professionals with experience in data extraction, transformation, and loading. DP-700 is designed for professionals who need to create and deploy data engineering solutions using Microsoft Fabric for enterprise-scale data analytics. Learners should also have experience at manipulating and transforming data with one of the following programming languages: Structured Query Language (SQL), PySpark, or Kusto Query Language (KQL).
About this Course
Course Outline
Implement and manage an analytics solution (30–35%)
Ingest and transform data (30–35%)
Monitor and optimize an analytics solution (30–35%)
Implement and manage an analytics solution (30–35%)
Configure Microsoft Fabric workspace settings
Configure Spark workspace settings
Configure domain workspace settings
Configure OneLake workspace settings
Configure data workflow workspace settings
Implement lifecycle management in Fabric
Configure version control
Implement database projects
Create and configure deployment pipelines
Configure security and governance
Implement workspace-level access controls
Implement item-level access controls
Implement row-level, column-level, object-level, and file-level access controls
Implement dynamic data masking
Apply sensitivity labels to items
Endorse items
Orchestrate processes
Choose between a pipeline and a notebook
Design and implement schedules and event-based triggers
Implement orchestration patterns with notebooks and pipelines, including parameters and dynamic expressions
Ingest and transform data (30–35%)
Design and implement loading patterns
Design and implement full and incremental data loads
Prepare data for loading into a dimensional model
Design and implement a loading pattern for streaming data
Ingest and transform batch data
Choose an appropriate data store
Choose between dataflows, notebooks, and T-SQL for data transformation
Create and manage shortcuts to data
Implement mirroring
Ingest data by using pipelines
Transform data by using PySpark, SQL, and KQL
Denormalize data
Group and aggregate data
Handle duplicate, missing, and late-arriving data
Ingest and transform streaming data
Choose an appropriate streaming engine
Process data by using eventstreams
Process data by using Spark structured streaming
Process data by using KQL
Create windowing functions
Monitor and optimize an analytics solution (30–35%)
Monitor Fabric items
Monitor data ingestion
Monitor data transformation
Monitor semantic model refresh
Configure alerts
Identify and resolve errors
Identify and resolve pipeline errors
Identify and resolve dataflow errors
Identify and resolve notebook errors
Identify and resolve eventhouse errors
Identify and resolve eventstream errors
Identify and resolve T-SQL errors
Optimize performance
Optimize a lakehouse table
Optimize a pipeline
Optimize a data warehouse
Optimize eventstreams and eventhouses
Optimize Spark performance
Optimize query performance
Duration
4 Days
Level
Intermediate